Blog
How Data Science Helps Threat Intelligence Analysis
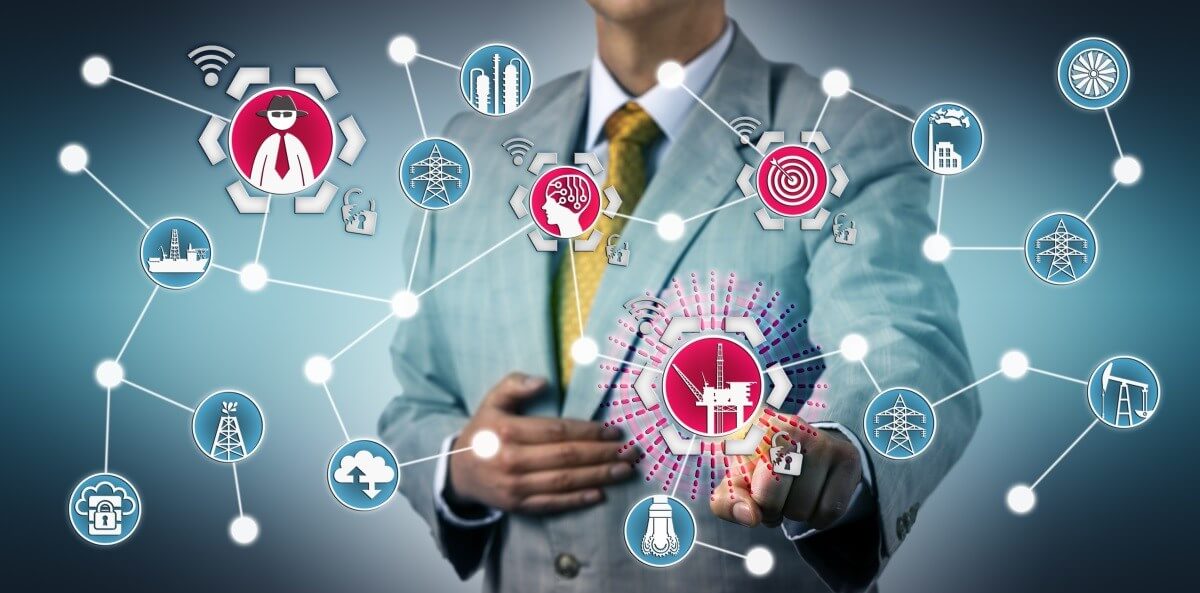
Data science is a major player in today's industries. Its valuable insights in a world powered by information are helping global businesses innovate, expand, and vastly improve. Threat intelligence is one arena where the integration of data science has offered a myriad of powerful benefits.
More and more threat intelligence teams are utilizing data science in their workflows. Helping analysts make better-informed decisions, data science is greatly expanding the power of threat intelligence. The uses of artificial intelligence (AI) are many and while it can't entirely replace human involvement in the process, it still offers a powerful tool in the battle against cybercrime.
Today’s analysts are faced with daunting tasks like evaluating thousands of datasets from various feeds and sources. The goal is to find insights, patterns, and trends within the data to identify malware campaigns or other deviant behavior that could indicate a threat. The entire process, however, takes a tremendous amount of time and effort for humans, making it inefficient.
Why Data Science is a Hero in Threat Analysis
Automation is valuable in many areas. When it comes to threat intelligence analysis, the ability to analyze, detect patterns and make solid predictions by using data from a proven automated process is a huge benefit. There are several facets within threat intelligence workflows where data science plays a vital role.
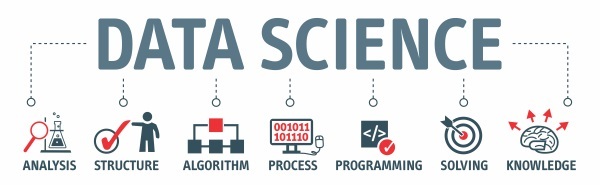
Automated Analysis
A huge task that AI does infinitely better and faster than humans, the automatic analysis of large sets of data greatly facilitates the work for the human analyst. Customarily collected in real time so analysts know the information is current, the data can be structured and timed as the analyst wishes and can reveal present moment indicators. With the help of machine learning, they may also be able to send classification or predictive reports.
Data Collections
In order to carry out any of their operations, like spreading malware, threat actors must create data points. Experienced analysts are able to craft a collection utility to track all C2 commands sent by the threat actor.
Text is also a prevalent data point. Since the internet is the venue exploited by most threat actors, the individual platform used as a mode of communication is another communication route for data collections. An entire team might be centered around collection, while another team could focus solely on data engineering. They work together to move and process data through the different stages of automated analysis.
Machine Learning
Machine learning involves some risk-taking because the data collection is dependent on how the results are interpreted. Computers are trained to interpret information without being specifically instructed on how to process it. When it comes to threat intelligence using data science, the resulting data can offer comprehensive, highly useful insights above what keyword searches and automated mathematics could produce.
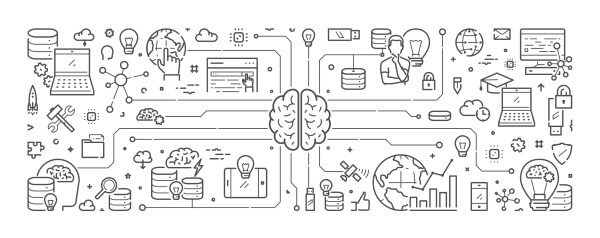
When developing machine learning processes for threat intelligence analysis, the subject matter expertise of experienced analysts is crucial. Analysts work with machine learning engineers to craft accurate, exact models with great specificity because when it comes to determining results, domain expertise cannot be underestimated. In instructing a machine to look for and comprehend certain aspects of the cyber threat landscape, analysts benefit from an increased understanding of threat actors, the detection of threat and trend variances, and insights into when a certain malicious event may happen.
Key Processes
Depending on a given issue, there are several methods data scientists may utilize to produce insightful analyses.
Knowledge Graphs
Visual representations of cyber threat analysis, knowledge graphs use edges and nodes like those used in graph theory. Nodes depict the various threats or events while the edges show the defined relationship between them. These are often very useful in prompting further questions and, if fed with new data, can be refined and remodeled to be of great use in identifying threats of all types.
Natural Language Processing
With the scope of text data generated daily, natural language processing (NLP) is being used with increased frequency by threat intelligence teams. With cybercrime being committed in all corners of the globe, being mostly facilitated through online communications, NLP machine learning analysis can prove very useful. There are several NLP methods used by threat intelligence teams like topic modeling.
Probability and Statistics
Simple tools are often the most effective ones. Moving averages can be used to understand the trends of threat points in a time series like mentions of a specific virus or piece of malware in particular forums previously. Further analysis can take the trace further in detecting and comprehending the aftermath of given cyber events.
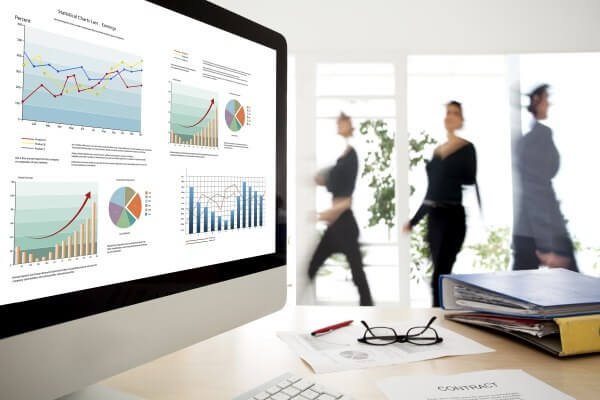
Supervised and Unsupervised Techniques
In the cyberthreat arena, analysts must be ready to get involved when a threat is predicted. The level of involvement will depend on the machine learning technique that made the prediction. If it was an unsupervised process, the results are latent and can offer not a definitive answer but rather a scenario that's more subjective and left to the interpretation of the analysts. Supervised methods offer forecasts within a discrete target label space and the results don't require a ruling. Machine answerability is still vital here.
The Future of Data Science in Threat Intelligence
Threat intelligence using data science will only continue to grow, which is a good thing given the rate at which cybercrime is growing. Data scientists are developing many comprehensive tools to help businesses detect threats so they can develop solid plans of action and better protect themselves.
Suites of APIs, services, and tools like those offered by Threat Intelligence Platform can provide businesses with optimal threat detection and comprehensive analysis at reasonable prices. When one factors in the potential cost of a potential cybercrime attack on a business, investing in strong preventive measures make sense.
Thanks to the exponential growth of AI, we can manage the growing crush of data produced each day. It better equips data scientists in their fight against cybercrime so they can help businesses protect themselves.
Read the other articlesHave questions?
We work hard to improve our services for you. As part of that, we welcome your feedback, questions and suggestions. Please let us know your thoughts and feelings, and any way in which you think we can improve our product.
For a quick response, please select the request type that best suits your needs.
Threat Intelligence Platform uses cookies to provide you with the best user experience on our website. They also help us understand how our site is being used. Find out more here. By continuing to use our site you consent to the use of cookies.